Discover the Best Train Journeys Around the World
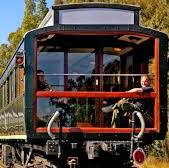
The Best Train Journeys Around the World Travelling by train offers a unique and scenic way to explore some of the most beautiful destinations in the world. From winding through lush countryside to crossing breathtaking mountain ranges, train journeys provide an unforgettable experience for travellers seeking adventure and relaxation. One of the best train journeys […]